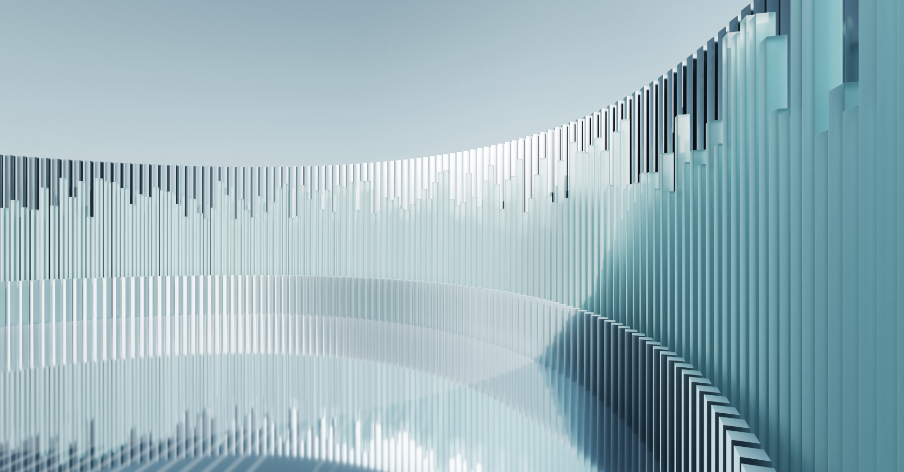
Refract: An Overview
Refract is a DSML platform that helps multiple personas like Data Scientist, ML Engineer, Model Quality Controller, MLOPs Engineer, and Model Governance Officer work seamlessly together on any AI use case. Refract accelerates each stage of the ML lifecycle, including data preparation, model development, model deployment, scoring, and monitoring on snowflake data.
Large Language Models — LLMs
Large Language Models are advanced artificial intelligence models that have been trained on vast amounts of text data to understand and generate human-like text. These models are designed to process natural language input, enabling them to understand and generate response text in a way that is contextually relevant and coherent.
LLMs, such as GPT-3 (Generative Pre-trained Transformer 3) developed by OpenAI, have achieved significant advancements in natural language processing and have been applied to a wide range of tasks, including text completion, language translation, question- answering, and more. They can understand and generate text in various languages and can be fine-tuned for specific applications and domains.
The OpenAI API is a cloud-based service provided by OpenAI, where you make requests to OpenAI’s servers to access the language models and receive responses — you do not host the API infrastructure or models on your own servers. But the APIs can be integrated into your applications or services by making HTTP requests to the OpenAI servers.
Limitations of Cloud-hosted private LLMs
Let us discuss a couple of limitations of such Cloud- hosted LLMs.
- Data privacy and security: The user might need to send input data (that might be sensitive) to cloud servers (where the LLM is hosted) for processing, and this may be in direct violation of enterprise compliance with relevant data protection regulations.
- Lack of transparency: Due to the intricate nature of such models, comprehending them can prove difficult for individuals. The lack of transparency further compounds the issue, impeding one’s understanding of how these models handle data and utilize information for decision-making. Consequently, this could result in limited transparency, and potential issues related to safety and privacy.
Solution: Self-hosted, open-source LLMs
If you look at the timeline (see image above), you will find that several of these LLMs are a part of the open-source community. Usually, these open-source models are released with some pre-trained weights, after getting trained on generic data such as Wikipedia’s crowd-sourced content. These models can either be directly deployed for consumption or they can be fine-tuned on custom data before deployment.
As the title of this blog suggests, the open-sourced models can be fine-tuned/trained on Refract and deployed in Snowflake.
In this blog, I will provide a clear guide on how to fine-tune a T5 model on Refract for text summarization. In this example, we make use of PyTorch and HuggingFace transformers for fine-tuning the model on a News dataset. After fine-tuning, the model was able to generate a summary of long news content.
In this blog we will also illustrate how to deploy the model as a Python UDF (User Defined Function) on Snowflake.
Step-by-Step Guide:
Step 1: Create a project on Refract and attach your GitHub repository to it. This will help in managing all your code.
Step 2: Open the project and launch a template for writing the code. Refract offers multiple pre-designed templates, including one specifically for Snowflake. This template is designed with all the necessary dependencies for Snowflake and flexible to run on various compute options, including GPU, CPU, or Memory Optimized instances. Once you’ve made your choice, you can launch the corresponding template and get started with your Jupyter Notebook right away. In our case of fine-tuning an LLM, we will opt for the GPU enabled Snowflake template.
Step 3: Import libraries and set PyTorch device to GPU. Fine-tuning is a compute-intensive operation, this step enables Refracts GPU to accelerate fine-tuning by parallelizing tasks.
Step 4: Use the following class to initialize the dataset. This will help in tokenizing and preparing the data for fine-tuning. The data should have two columns: one is the document of arbitrary length, and the other is its shorter version or summary.
Step 5: Add the following two functions for training and evaluation.
Step 6: Load the T5 model. T5, an encoder-decoder model, operates by transforming all NLP problems into a text-to-text format. During training, it utilizes a technique called teacher forcing, which necessitates an input sequence and a corresponding target sequence. The input sequence is provided to the model using input_ids, while the target sequence is modified by shifting it to the right. This involves adding a start-sequence token at the beginning and feeding it to the decoder via decoder_input_ids. In the teacher-forcing approach, the target sequence is extended with an EOS token and serves as the label. The start-sequence token is represented by the PAD token. T5 offers the flexibility of being trained or fine-tuned in both supervised and unsupervised manners. In our case we will fine-tune the model in a supervised manner.
Step 7: Prepare training and validation data loaders.