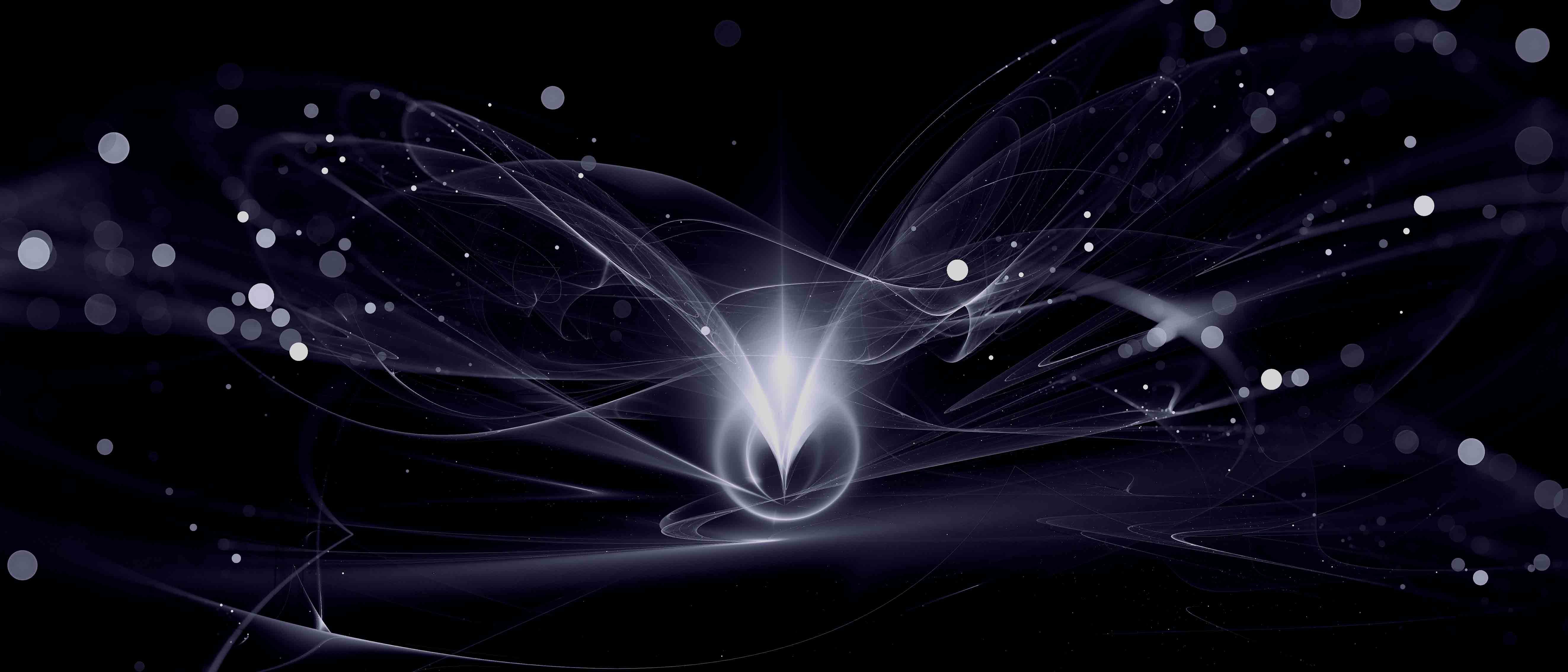
Introduction
At the heart of data science and analytics lies the critical role of features, acting as a cornerstone for precise decision-making and the development of predictive models. Feature refers to an individual, measurable property or characteristic of the data that is used as input for machine learning models. Despite organization’s earnest efforts to unlock the latent potential within their raw data, expectations often need to be managed. This narrative unfolds as we delve into the powerful capabilities of Snowflake and Refract, the Fosfor Decision Cloud’s Insight Designer, understanding how their seamless combination untangles the complexities and elevates data and feature management to new heights.
Challenges in feature management
Building and managing features in an ever-evolving landscape of data-driven decision-making can be daunting, as it involves navigating various challenges. While features play a vital role in providing valuable insights, organizations often encounter obstacles while trying to leverage them to their full potential. These challenges put the data teams to the test and highlight the need for intelligent solutions. In this context, it is essential to explore the difficulties of managing features and understand the most common problems organizations face while trying to unleash the power of their data.
- Limited Data Lineage tracking – Data Lineage is the line of information, unveiling your data’s origin, transformations, and destinations. The absence of Data Lineage hampers transparency and accountability. It becomes exceptionally crucial in feature management, where the origin and evolution of features play a pivotal role in data-driven initiatives. This constraint in tracking Data Lineage can impede transparency, accountability, and the ability to make informed decisions.
- Lack of version control – The need for version control introduces a significant challenge. Version control is equivalent to a safety net, ensuring that every iteration, modification, or feature enhancement is systematically documented and accessible. Without this safeguard, organizations face the risk of versioning chaos, where it becomes difficult to track changes, replicate successful models, or maintain consistency across different stages of feature development.
- Collaboration bottlenecks – The bottleneck emerges when data engineers offer inconsistent or low-quality data for feature creation, causing difficulties for data scientists and ML engineers in constructing reliable models. This issue is exacerbated by the isolated work of data scientists and ML engineers during the feature engineering phase, leading to duplicated efforts, potential inconsistencies in feature extraction, and delays in model development.
- Data quality and consistency – The accuracy of features hinges on ensuring error-free data derivation, as inaccuracies can compromise insights and decision-making. Comprehensive documentation and metadata detailing the data used for feature creation foster transparency and understanding among team members. These practices are indispensable for organizations seeking reliable and impactful feature management, ensuring the robustness and effectiveness of their data-driven initiatives.
- Difficulty in model deployment – Integrating features and models into production is intricate, particularly when facing disparities between development and deployment environments. Challenges arise in deploying models at scale due to resource and scalability considerations, impacting the optimization of feature-driven models across diverse use cases. Real-time updates pose additional challenges, requiring prompt reflection of changes without disrupting ongoing processes.
Unlocking new possibilities in feature management with Snowflake and Refract, the Fosfor Decision Cloud’s Insight Designer
Exploring feature management challenges reveals that integrating databases and purpose-driven products is a catalyst for overcoming hurdles, streamlining processes, and enhancing efficiency. Snowflake’s Snowpark, a dynamic database, seamlessly integrates with Refract, a purpose-driven product, forming a powerful alliance that unlocks new possibilities in feature management, data processing, and insights generation.
Snowflake’s Snowpark
In the arena of feature management and database operations, Snowflake’s Snowpark emerges as a game-changing tool, enhancing the capabilities of the Snowflake cloud data platform. It allows for the seamless integration of personalized code, utilizing languages like Java and Scala, directly into the Snowflake environment. This functionality goes beyond traditional data processing, empowering users to address specific challenges related to feature management and database tasks.
Refract, the Fosfor Decision Cloud’s Insight Designer
Refract, an enterprise ML platform, consolidates top ML frameworks and templates to facilitate the preparation, construction, training, and deployment of high-quality Machine Learning (ML) models. This platform ensures a smooth and personalized “Build-to-Run” transition in AI workflows, reducing user effort by up to 70%. It expedites data science, AI, and ML life cycles through no-code automated features, significantly cutting down time and effort on various pre- and post-model development steps, including data provisioning, preparation, managing features, model deployment, governance, monitoring, and more.
Exploring the impact of Refract and Snowflake-Snowpark Integration
Step 1 Enable a suitable role for creating a feature store project
Refract is committed to fostering a cohesive and unified approach, promoting seamless collaboration among data scientists, data engineers, and machine learning engineers within our feature store framework. This commitment is driven by the dual goals of achieving comprehensive end-to-end traceability and facilitating agile and swift iterations across machine learning development stages.
Step 2 Establish a specific use-case context
Creating a use case to predict Customer Lifetime Value. Customer Lifetime Value (CLV) aims to assess the total predicted value a customer is expected to bring to a business over the entire duration of their relationship. CLV is a strategic metric that focuses on understanding and maximizing the long-term revenue potential of each customer.
Step 3 Integrate your feature repository with Refract for centralized management
Refract seamlessly integrates with GitHub, providing a familiar environment for managing and versioning your feature repository. This integration ensures a smooth transition and enhances collaboration across teams.
Step 4 Connect to Snowflake to create an offline/online store
Ensure you have a Snowflake account and the necessary credentials to access your Snowflake instance. If you don’t have an account, sign up for Snowflake and create the required user roles and permissions.
Step 5 Configure your feature repository.
Click on “Configure feature store” to get started.
Enter the basic details and select the file.
Post configuring the feature store repository into Refract, proceed with the selection of offline and online configurations. Ensure you add the Snowflake connection details that you created.
And configure the frequency for materialization.
Your feature store is ready to get started. The project comprises of feature view, feature service, entity, and jobs.
Step 6 Overview of feature view
Feature view refers to a user interface or representation that provides a comprehensive overview of the features stored within the feature store.
Entities help organize and structure the features, which are the characteristics or properties of these things. So, when you retrieve features from a feature store, you’re essentially getting information about specific entities in your data, allowing you to understand and analyse their attributes.
This page consists of a type of database used to create the feature store. This database serves as a central hub for storing and retrieving features used in machine learning and data science applications. It typically includes mechanisms for version control, metadata storage, and efficient retrieval of features for analysis or model training.
Step 7 Overview of feature service
Feature service typically refers to a mechanism or interface that allows users, applications, or machine learning models to interact with and retrieve features from the feature store.
This screen provides users with all the feature views as a part of the feature service.
Step 8 Spin up any notebook
Refract allows the user to create custom coding environment by creating new templates.
Through this step users can launch an empty template to set up a notebook environment.
Now users can read the feature store project into the new notebook to fetch the historical data.
Users can also fetch the historical data by creating the entity dataframe.
Fetch the feature service from the feature store and use the entity dataframe.
This step involves creating new features or transforming existing ones to enhance the performance of a model.
Here we are training a model using Random Forest to predict customer lifetime value.
Now finally we are registering the model in Refract as shown below.
Step 9 Deploy the model
Here we are deploying the model in Refract. Implement your machine learning model into a production environment, making it operational for real-world use, and enabling it to generate predictions or insights as intended.
Once the model is deployed, users can seamlessly measure the metrics of the deployed model.
Conclusion
This blog sheds light on the critical aspects of feature management within the dynamic landscape of data-driven environments. The collaborative synergy between Snowflake and Refract, the Fosfor Decision Cloud’s Insight Designer emerges as a powerful solution to address the challenges associated with feature engineering, storage, and utilization. By leveraging Snowflake’s robust data platform and Refract’s feature management capabilities, organizations can establish a seamless and efficient workflow. The integration provides a centralized repository for features, ensuring end-to-end traceability, rapid iterations, and a cohesive strategy across the entire data science and machine learning lifecycle.