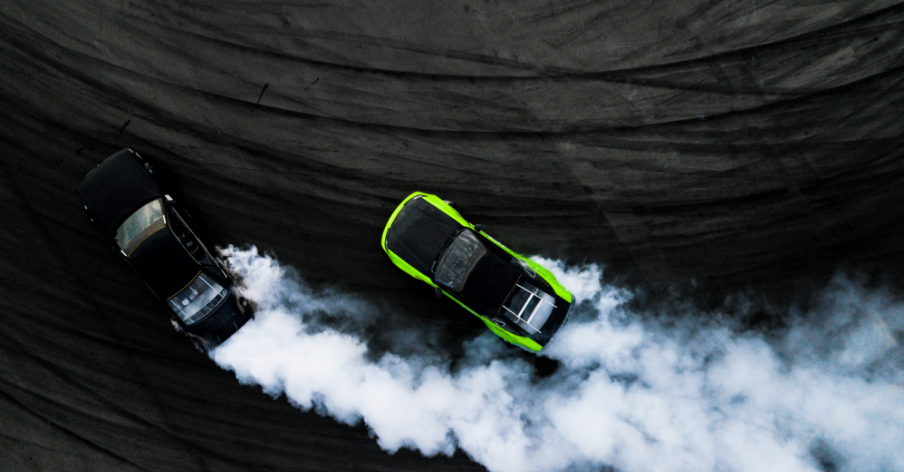
The only constant in life is change.
– Heraclitus.
The COVID-19 pandemic disrupted the world and caused outstanding issues. These include the shortage of beds in hospitals, the spike in the necessity of medical professionals, the Great Resignation, increases in poverty, surges in demand for daily resources, closed international borders and much more.
This rapidly evolving environment presented an unprecedented challenge to how businesses operate today. We can see this change by comparing past data with current data for most business scenarios.
Unsurprisingly, the data gathered from this time and the Machine Learning (ML) Models trained on these data shifted. This shift in the underlying behavior of ML technology is called drift.
Before their release, ML models are usually trained with very well-analyzed data. They are controlled by cleaning, carefully eliminating, and engineering the data they ingest. However, once the model is live in production, the model is exposed to real-world data, which tends to be dynamic and bound to change with time. This exposure leads to a gradual or sudden decay in model performance or metrics. This loss of model prediction power is called model drift.
Why does Model Drift occur?
There can be potentially three reasons for Model Drift in machine learning.
- 1. When the underlying behavior of the data changes due to some external events
These data changes can include events like recession, war, pandemics, etc. For example, during and post-COVID-19, people’s preferences and capacities for movement changed suddenly. A steep increase in the desire for personal immunity led to increased demand for natural/artificial immunity booster products.
- 2. When something goes wrong with the ML production setup, like a minor upgrade or enhancement
Errors at the ground-level lead to a change in incoming data values. For example, a slight change in a website allows the end user to enter the age manually rather than from predefined drop-down values without proper boundary checks for Null/Max values.
- 3. When the model’s training and application are misaligned
In this case, the ML model is trained for one context and applied incorrectly in a different context. For example, an Natural Language Processing (NLP) model trained on a corpus of Wikipedia data is used for writing news articles. This is not a fitting match because of the risk of utilizing inaccurate data.
Model Drift timeline
Once the model is deployed in production, model drift can also be categorized based on the pattern of time and magnitude.
Gradual Model Drift
A classic example is a change in the behavior of fraudulent customers trying to beat the Artificial Intelligence (AI)-based anti-money laundering systems. Simply put, as fraudsters become more vigilant of the existing AI/ML detection rules, they evade the system by changing their strategies to fool the model. Thus, the model quality will degrade as more and more fraudsters try to change their strategy over time.
Sudden Model Drift
When there is a sudden change in consumer behavior, the model trained on historical data won’t be able to account for the impact. During the pandemic, people started spending more on over-the-counter drugs for colds and coughs than earlier. Thus, a forecasting model trained on pre-pandemic data won’t be able to capture this underlying scenario.
Recurring Model Drift
This is more like drift seasonality. There is a behavior change, but it is repetitive or recurring. While analyzing the historical data for any online retail like Flipkart or Amazon, there is always a surge in the number of orders placed during festive sale seasons. When this data is fed to the model, it will lead to a drift in production.
Incremental Model Drift
When the occurrence of drift is continuous, but the magnitude increases with time, it is classified as incremental. A possible example could be a view time on viral advertisement content. Here with time, the average predicted view time would gradually shift away from the actual view time for a given week or month.
Blips in the Model Drift
You can think of blips as more of a noisy pattern where the drift is more random in terms of time interval and magnitude.
Fig 1 – Drift classification based on speed and magnitude
Types of Model Drift
Drift generally implies a gradual change over a period of time. The change in data or the relationship between predictors and the target variables drift are further categorized as given below.
Data Drift
Data drift is defined as a change in the distribution of data. In ML terms, it is the change in the distribution of scoring data against the baseline/training data. This can be further broken down into two categories.
-
1. Feature Drift
Models are trained on features derived from input or training data. When the statistical properties of the input data change, it might have a domino effect on the model performance and business Key Performance Indicators (KPIs). This can happen due to changes in trend, seasonality, preference changes, gradually with time or due to some uncontrolled external influencing factors. This deviation in the data during the overall time to market is called feature drift.
Fig 2 – Illustrating the model’s time-to-market journey
Additionally, failing to identify drift before model deployment may lead to a severe negative impact. Recommending a wrong playlist to a user is less harmful than suggesting a lousy investment option. This can lead to business or brand impact. To mitigate this risk, data drifts can sometimes be handled by simply retraining the existing model with the latest data. Sometimes one may have to replan everything from scratch or even scrap the model.
-
2. Label Drift
Label drift indicates that there is a change in the distribution of model output. In a loan defaulter use case, if the approval ratings are higher than the testing output, this would be an example of label drift.
Fig 3 – Explains the concept of Feature and Label drift with the decision boundary
Concept Drift
Concept drift occurs when there is a change in the relationship between the input and output of the model. Consider that a model, once trained, establishes a relationship P(Y/X) between an Input feature (X) and Output label (Y). Concept drift is further classified into two categories:
-
1. Virtual Drift:
When there is a change in the distribution P(Y/X) of the Model input and true label, but the model performance still hasn’t changed.
-
2. Real Drift:
When there is a change in the distribution of the Model input and true label, resulting in a change in the model performance.
Fig 4 – Illustrates Virtual drift v/s Real drift
For a loan application example, people often look at the loan applicant’s age, background checks, salary, loan history, credit ratings, etc. Age is often considered an essential feature (of higher weightage) when fed to the loan processing AI application. However, during the pandemic, the government announced several measures to curb the interest rate on home loans. This resulted in many older people also starting to apply for loans. This change in the behavior tends to decay the model unless it is retrained to capture the change in relationship to make it more robust to the current market scenario.
Fig 5 – Shows the effect of model retraining on the model accuracy
Prediction drift
Prediction drift is a deviation in a model’s predictions. The change in the model prediction distribution over time depicts the prediction drift. If the distribution of the prediction output label changes significantly, the underlying data will most likely change significantly. For example, over a given period, the prediction becomes more skewed towards a particular class label though there is no model decay.
Drift handling
Retraining the model with current data or additional labels is always a safe option for handling drift. However, given the time and cost involved, this might not always be the right approach.
Usually, it is best to find the root cause by checking the training features, comparing the distribution pre- and post-deployment, analyzing the label distributions, and a few more.
Piotr (Peter) Mardziel’s blog on Drift in Machine Learning explains that these training features include the following: