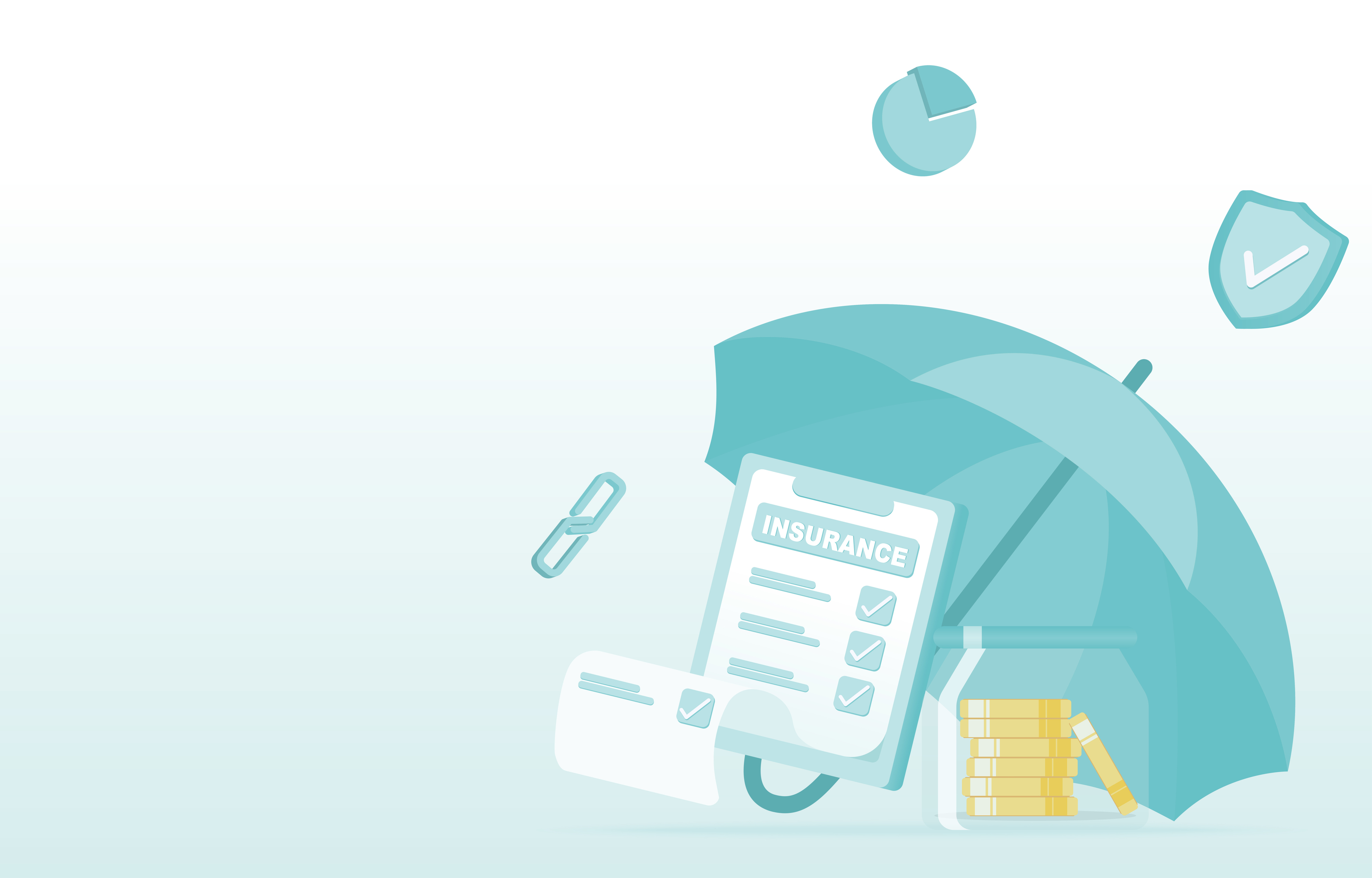
Introduction
Claim reserve optimization stands at the core of the insurance sector, underscoring its crucial role in ensuring financial stability and adherence to regulatory mandates. This intricate process involves determining the necessary financial reserves to settle future claims, thus directly impacting on an insurer’s liquidity and solvency. Traditionally, this task relied on historical data and actuarial techniques, which, while effective, often fell short in accuracy and timeliness. The reliance on past data without the ability to analyze real-time inputs or predict future trends introduces significant challenges, limiting the effectiveness and efficiency of reserve estimation.
The evolution of traditional claim reserve methods
The journey from traditional methodologies towards more advanced approaches in claim reserve estimation reveals a landscape marred by inefficiencies and inaccuracies. In the past, insurance companies relied heavily on traditional claim reserve methods to estimate future payouts for claims. Manual calculations and estimations, hallmarks of the conventional process, not only consume considerable time but also introduce a margin of error that could have substantial financial implications. This approach, characterized by its static nature, struggles to keep pace with the dynamic and fast-evolving demands of today’s insurance market, highlighting the pressing necessity for a paradigm shift towards more dynamic, accurate, and efficient reserve management strategies.
As a result, the industry started exploring alternative approaches that leverage advanced data analytics, machine learning algorithms, and predictive modeling techniques to improve reserve estimation accuracy and efficiency. These modern methods not only offer real-time insights into claim liabilities but also enable insurers to proactively manage risks and optimize their capital allocation. As technology advances, the evolution of claim reserve methodologies remains a critical area of focus for insurers seeking to enhance their competitiveness in an increasingly dynamic marketplace.
The transformation through AI
The advent of Artificial Intelligence (AI) has heralded a new era in claim reserve optimization, marking a departure from traditional methodologies towards more accurate, swift, and predictive analytics-driven approaches. AI algorithms, with their capacity to digest vast datasets and uncover hidden patterns, offer unprecedented foresight into future claims and optimal reserve levels. This capability significantly transcends the limitations of traditional methods, introducing a level of precision and efficiency previously deemed unattainable. Through AI, insurers can now forecast with greater confidence, ensuring financial reserves are both adequate and in alignment with future liabilities.
Harnessing data with ELT
The Extract, Load, Transform (ELT) methodology serves as a pivotal foundation in the data preparation phase, crucial for the successful application of AI in claim reserve optimization. This approach allows for the seamless aggregation, cleansing, and enrichment of data from a plethora of sources, rendering it primed for in-depth analysis. By streamlining this preparatory phase, ELT not only enhances the quality and accessibility of data but also significantly expedites the process, setting the stage for AI and analytics to efficiently generate insightful and accurate reserve estimations.
AI methodologies in predictive modeling
AI methodologies, including machine learning and deep learning frameworks, stand at the forefront of predictive modeling for claim reserve optimization. These sophisticated technologies harness the power of historical and real-time data to identify patterns, predict future claims, and accurately assess risk levels. The application of such AI-driven models enables insurers to precisely calculate reserve requirements, thereby optimizing financial resources and mitigating potential risks. This evolution from heuristic-based estimations to data-driven predictions represents a significant leap forward in the quest for accuracy and efficiency in reserve management.
Applying decision intelligence in managing claim reserves
The incorporation of Decision Intelligence techniques, such as Diagnostic Analytics, Prescriptive Analytics, and Natural Language Generation (NLG), further refines the process of claim reserve optimization. These methodologies provide a comprehensive understanding of data, facilitating informed decision-making by interpreting complex datasets, recommending action plans, and generating intelligible reports through natural language querying. This multifaceted approach not only enhances the precision of reserve estimations but also democratizes the decision-making process, enabling stakeholders to make more informed, strategic choices.
The role of the Fosfor Decision Cloud (FDC)
The FDC is an end-to-end, comprehensive decision intelligence platform designed to facilitate the entire data-to-decision process in claim reserve optimization. This platform seamlessly integrates data management, AI-driven analytics, and decision intelligence techniques, empowering insurers with actionable insights and optimized reserve levels.
- The FDC’s Data Designer – Insights are only as effective as the data they are based on, and good quality data depends on how well it is managed. The Data Designer simplifies the development and maintenance of data transformation pipelines which empowers efficient data ingestion, error-free transformation, and continuous pipeline health monitoring for reliable insight generation while ensuring data transparency and traceability. This facilitates real-time access to critical insurance data, empowering stakeholders to make timely and informed decisions.
- The FDC’s Insight Designer helps enterprises build, train, deploy, and manage AI models at enterprise scale. It is a centralized, scalable, and collaborative environment for building your ML/DL/Large Language Models using your language and IDE of choice. It takes care of technical infrastructure and scalability thanks to auto scaling, on-demand resource allocation, distributed computing, in-database analytics, and support for both GPU and distributed training frameworks.
- The FDC’s Decision Designer allows you to leverage the power of AI to explore your data and model output to get instant answers, get alerted to interesting changes in your data, and access your insights anywhere, enabling more timely, impactful outcomes. By articulating questions in plain English language, insurers can receive instant responses with actionable insights, eliminating the need for complex querying/technical expertise.
The capabilities and advantages of the FDC extend beyond mere data processing, offering a holistic solution that addresses current challenges and ushers in a new era of efficiency, accuracy, and strategic decision-making in insurance financial management.
Embracing the future with the FDC
The journey towards optimizing claim reserves is emblematic of the broader transformation within the insurance industry, underpinned by the adoption of AI and decision intelligence. The FDC emerges as a beacon of innovation in this landscape, offering a comprehensive solution that transcends traditional methodologies. By harnessing the power of data, AI, and advanced analytics, insurers are now equipped to navigate the complexities of claim reserve optimization with unprecedented precision and efficiency. Embracing the FDC signifies a commitment to leveraging cutting-edge technology for enhanced financial stability, regulatory compliance, and strategic decision-making.