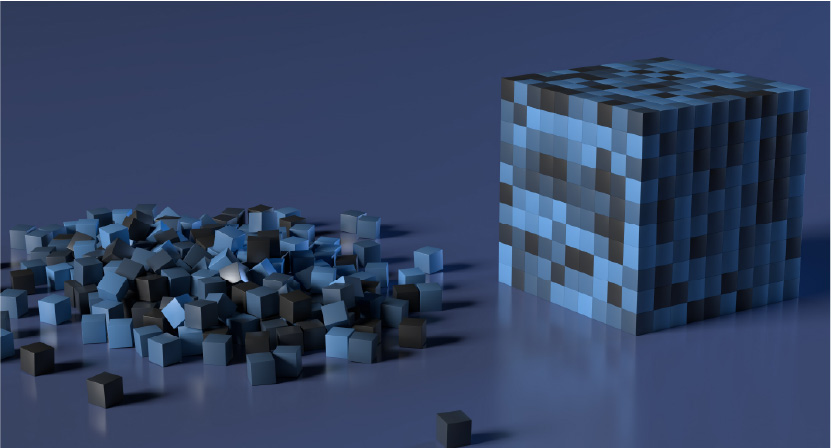
In today’s data-driven world, the ability to transform and integrate data efficiently is crucial for businesses seeking valuable insights. Data transformation is a key process in the data lifecycle, converting raw data into a format suitable for analysis and decision-making. As organizations move their data to the cloud, the demand for powerful cloud data integration tools and platforms has grown exponentially.
This article delves into the significance of data transformation, explores the features of our solution, and examines how it empowers data engineers to improve data quality and streamline cloud transformation workflows. By the end, readers will understand how this innovative tool is reshaping data engineering and cloud data integration.
Understanding data transformation
Definition and importance
Data transformation involves converting, cleansing, and structuring data into a usable format to fortify decision-making processes and propel organizational growth. It modifies data to fit a particular purpose or context, enhancing its quality and usability for accurate analysis. As companies collect data from multiple sources, compatibility becomes a concern, making data transformation increasingly important.
Organizations must consolidate, clean, and restructure data into a usable format for analysis. A successful transformation process enhances data quality, insights, accessibility, and security, supporting better decision-making. The goal is to create consistent, accessible data providing accurate analytic insights and predictions.
Key components of data transformation
The data transformation process typically involves several key components:
- Discovery: Understanding the source data’s type, structure, and quality. (Eventually)
- Mapping: Determining how individual data elements are translated from the source to the target system.
- Code generation: Applying transformation rules to convert data into the desired format.
Following these steps, common data transformation techniques are essential for refining and preparing data. Normalization scales data to a standard range, ensuring all variables contribute equally. Aggregation summarizes detailed data at a higher level, such as quarterly sales, helping identify broader trends. Generalization replaces specific values with more general ones, aiding in anonymization and reducing complexity. Attribute construction creates new attributes, like calculating customer lifetime value, offering new insights. Lastly, Data cleansing corrects errors and inconsistencies, ensuring data accuracy. Together, these techniques enhance data utility, making it more suitable for analysis and decision-making.
Common challenges in data transformation
Despite its benefits, data transformation presents several challenges:
- Data retrieval and storage: Organizations must design storage systems to accommodate rapid data growth and be prepared for significant scalability.
- Data security: As data volumes increase, so do risks of breaches and cyber-attacks. Robust security measures such as encryption, access controls, and data masking are essential.
- Data integration and interoperability: Breaking down data silos and integrating data into a unified system can be challenging. Solutions include data virtualization, data warehousing, and data governance.
- Data quality and accuracy: Maintaining high data quality can be challenging due to consistency issues, errors, and duplication. Establishing data governance policies and leveraging data quality tools can help.
- Compliance with privacy regulations: Organizations must comply with regulations like GDPR and CCPA by implementing robust data security measures and providing transparency about data usage.
- Legacy system migration: Moving data from outdated systems to modern databases often requires a comprehensive strategy including data profiling, cleansing, and validation.
- Talent and skills shortage: Skilled professionals with SQL knowledge or Python expertise are needed to manage and transform data effectively.
Organizations must invest in efficient data processing methods, choose appropriate storage solutions, and implement comprehensive data governance strategies to address these challenges.
Unless there is a different approach available – the Fosfor Decision Cloud’s Data Designer.
The Data Designer: An Overview
The Fosfor Decision Cloud’s Data Designer accelerates the construction of reliable, performant, and scalable data transformation pipelines. It enables organizations to curate AI-ready data, thereby speeding up decision-making processes. As the world is predicted to create approximately 463 exabytes of data each day by 2025, the need for a modern data infrastructure to store, transform, and extract meaningful insights has never been greater.
Key features and capabilities
- Flexible development environment: The Data Designer offers a low-code canvas for non-coders, allowing for the development of data pipelines that can be built once and deployed across various environments.
- Modular and optimized code: The platform produces modular and optimized code for push-down transformation, resulting in faster data processing and reduced credit consumption.
- Data reliability and monitoring: The Data Designer incorporates configurable data reliability rules, comprehensive monitoring, end-to-end lineage, and automated anomaly detection to prevent costly errors and improve data quality.
- Interactive GenAI assistance: The platform provides AI-powered assistance for constructing DBT models and data pipelines, helping users generate analysis on data cloud elements while organizing the data.
- Seamless cloud integration: Modern data pipelines built with the Data Designer can run on multiple cloud environments and data analytics engines, ensuring flexibility and avoiding vendor lock-in.
How it addresses common pain points
- Data organization and structuring: The Data Designer helps structure and organize data to optimize it for creating data products for analytics and AI application consumption.
- Efficient pipeline development: The platform enables the construction of efficient, scalable transformation pipelines that adhere to industry-standard software engineering practices.
- Data quality assurance: By offering configurable alerts, data reliability rules, and a comprehensive dashboard, the Data Designer helps identify and rectify data anomalies, ensuring overall data quality.
- Streamlined collaboration: The platform incorporates seamless, integrated version control and DevOps capabilities, facilitating streamlined collaboration and accelerating time-to-market for new pipelines.
- Enhanced productivity: With one click configuration and drag and drop features along with co-pilot it ensures faster and efficient building of data pipelines for anyone and everyone.
Enhancing data transformation with Fosfor
Streamlining the transformation process
The Fosfor Decision Cloud’s Data Designer offers a flexible low-code canvas, allowing for streamlining the pipeline development process and enhances productivity.
Improving data quality and reliability
The Data Designer incorporates several features to ensure data quality and reliability:
- Configurable data reliability rules
- Comprehensive monitoring
- End-to-end lineage
- Automated anomaly detection
These features help prevent costly errors and improve confidence in data quality. The platform provides a 360-degree view of the data ecosystem, offering unrivaled insights into pipeline status, data quality, and performance. Users can identify and rectify data anomalies through configurable alerts, data reliability rules, and a comprehensive dashboard.
Conclusion
The Fosfor Decision Cloud’s Data Designer is changing the game in data transformation, offering a powerful solution to tackle common challenges in the field. Its flexible development environment, data reliability features, and seamless integration with existing ecosystems enable organizations to build trustworthy data pipelines and make faster, more informed decisions. This tool not only streamlines the transformation process but also significantly impacts data quality and reliability, giving businesses the edge they need in today’s data-driven world.
Looking ahead, the future of data transformation seems bright with tools like the Fosfor Decision Cloud leading the way. As organizations continue to grapple with ever-growing volumes of data, solutions that can efficiently process, transform, and extract meaningful insights will be crucial. Fosfor’s approach to combining AI assistance with human expertise points to a future where data transformation becomes more accessible, efficient, and impactful, ultimately helping businesses thrive in an increasingly competitive landscape.