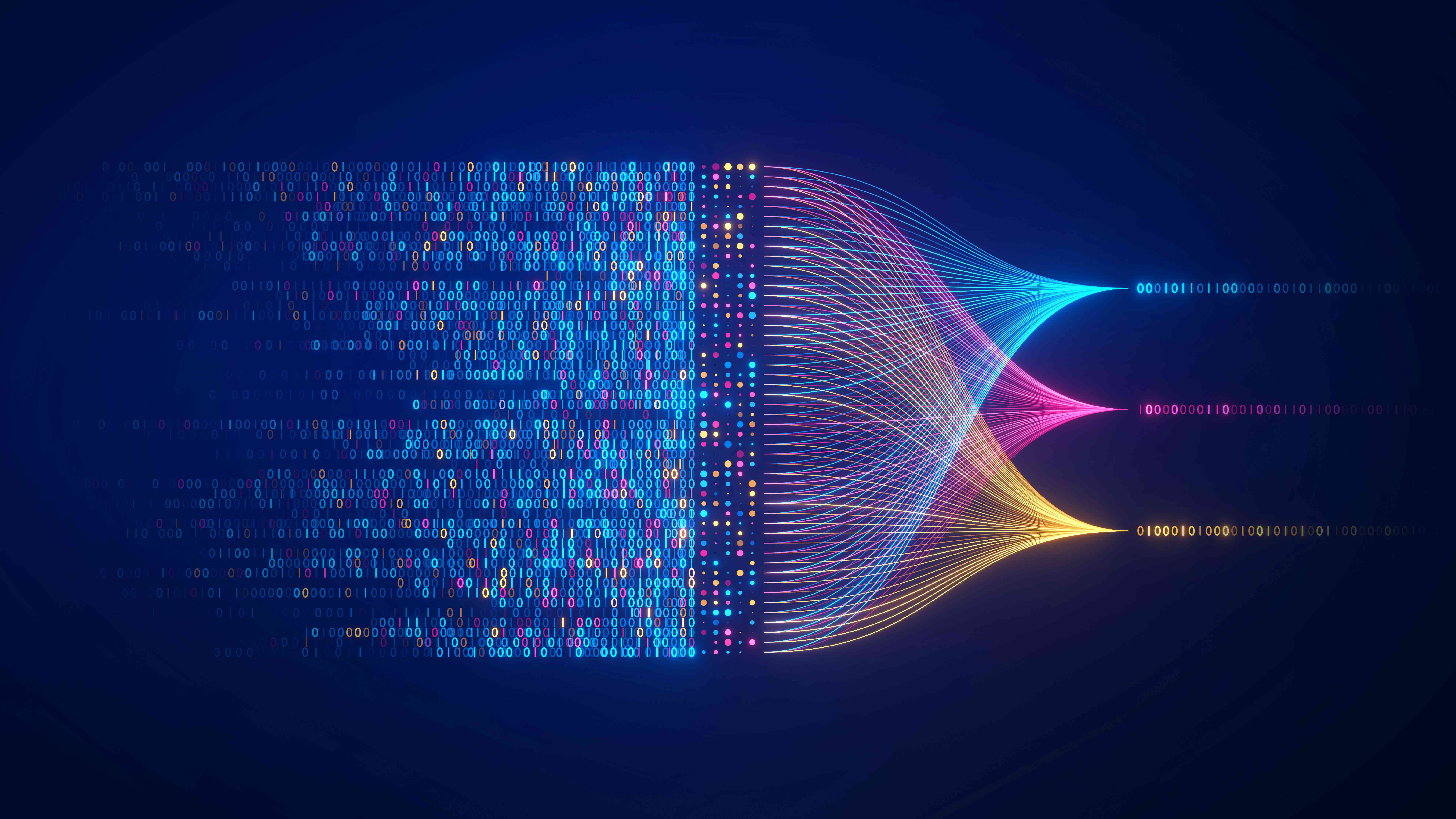
Part 1: The problem of plenty
Introduction
The promise of AI is irresistible – insights that can transform business decisions, increase efficiency, and give companies a competitive edge. However, the road from raw data to trusted data and insight, which can deliver meaningful business outcomes is often riddled with potholes. Based on challenges I’ve seen at multiple organizations, here are 5 common issues that disrupt the data-to-decisions journey.
Too many tools lead to a disjointed data-to-decisions journey
Today’s data technology landscape is crowded. From data ingestion to storage to analysis, there are multiple tools companies use to try to optimize different stages of the journey. However, this leaves customers stitching together disjointed solutions with increasing levels of complexity and frustration. Without a consolidated platform, the hand-offs between tools become brittle. Data context, security, and governance get lost in translation. The whole chain is only as strong as the weakest link so problems multiply across a complex environment. Customers spend most of the time connecting and integrating tools, not on transforming data to business outcomes.
Tools are overly complex to learn and use
In attempting to be everything for everybody, several tools within the data ecosystem have become overly complex trying to solve every edge case. There are zillions of features offering no definitive value, but simply adding to the cost & complexity of these tools. Learning and using these tools hence require specialized expertise and abundant time. Such “feature creep” or “feature bloat” not only increases costs but also hinders adoption.
Lack of ROI
Despite massive spending on data infrastructure, most companies struggle to demonstrate concrete returns on their investments made on AI. Businesses struggle to unlock the value hidden within their ever-growing data repositories. One key reason is that the costs to string together and maintain a complex data ecosystem outweigh the gains. The costs of storage, maintenance, and analysis quickly mount, while the promised return on investment remains elusive. There are several successful PoCs (Proof of Concepts) but most of them don’t get deployed into production. Of those that do get deployed, very little is used by end users.
Vendor lock-ins block future innovation
The complexity of today’s data technology landscape has resulted in solutions that are highly proprietary and locked into a single vendor’s stack. Once you settle on core components like storage, ETL, visualization, and analytics, you are trapped into that vendor’s ecosystem forever. This blocks companies from adapting new technologies as they emerge. Being able to integrate best-of-breed capabilities is key to avoiding lock-in. Open ecosystems built on common standards provide flexibility and interoperability that let you innovate into the future.
Lack of adoption
Often, companies make significant technology investments but fail to achieve adoption across their business users. Because of the fragmented data journey, users must piece together data context across tools. Complexity of use and maintaining data hygiene become serious responsibilities for business users. They end up avoiding the mess by making decisions on gut feel or based on rudimentary built-on-excel reports, rather than using data lakes or data warehouses. Low adoption eventually leads to low RoI.
Conclusion
In summary, companies continue to struggle with basic data management and insight creation steps, even today. Despite rapid innovation of data technologies, companies fail to translate this to increased productivity or business growth. The breakneck pace of the innovation and adoption of new tools, products, frameworks, and platforms has paradoxically created complexity and fragmentation. With so many options to evaluate, companies end up spreading their technology investments across disjointed products and pilots with no tangible gains. We seem to be drowning in an ocean of products and platforms with little hope of survival.
Or so it may seem, for now. In part 2 of this post, I will explain how we can solve this problem.