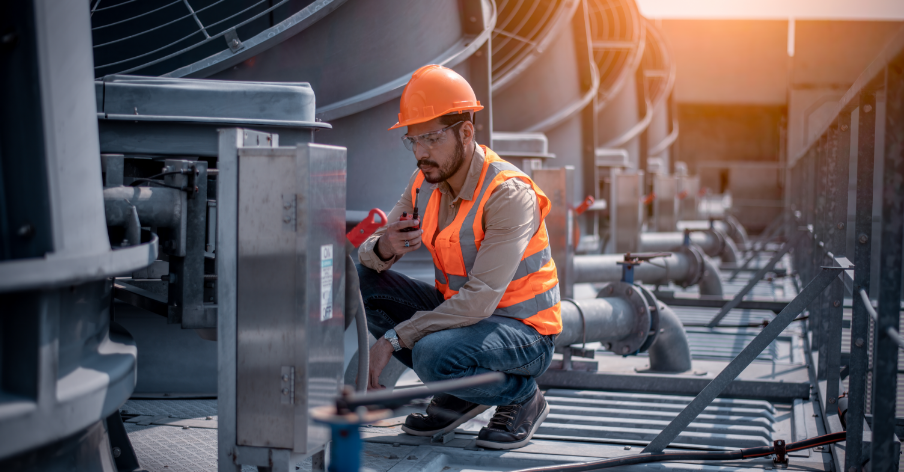
For manufacturers seeking to improve their financial performance and customer satisfaction, the quickest route to success is often a product-quality transformation focusing on reducing warranty costs. When fulfilled while backing exceptional product quality, product warranties positively impact sales, profitability, and customer loyalty. On the other hand, when warranties are backed by sub-optimal products, it results in large warranty reserves that directly impact the organization’s profitability. This negatively impacts the organization’s brand, Image, and equity.
Analyzing warranty claims: Why is it necessary?
Managing warranty claims (and spending) is an organization-wide challenge impacting multiple functions. These include:
- Quality
- Finance
- Product engineering
- Customer service
- Procurement
- Warranty administration
Considering the disparate objectives across functions, the warranty data is often looked at with inter-dependent functional data residing in disparate systems to derive actionable insights. This departmentalized approach to analysis forces the aggregation of data and information to a very high level. This state limits the ability to:
- Track, trace, and analyze (on a single pane of glass) disparate data in the warranty chain.
- Detect and avoid product failures before they occur.
- Accurately predict warranty cost obligations and reserves.
- Quickly identify the root cause of complex systems and reduce the detection to correction (DTC) time.
- Reduce fraud in the warranty chain.
- Identify the erroneous vendors/suppliers and drive recovery.
Fortunately for the business leaders tasked with managing and reducing warranty budgets, product recalls, assessing vendor quality, and supplier financial recovery, there is help in the form of Decision Intelligence platforms like Lumin.
The data
This blog post analyzes the warranty claims data for a global HVAC manufacturer using Lumin. In this blog, we will also highlight some of Lumin’s key capabilities in the process. These include:
- Proactively identifying anomalistic trends in sales value and subsequent diagnosis across channels, product hierarchy, pack type, variant, etc.
- Identification of the market share drivers and simulation of their impact on sales.
- Accurate prediction of the overall sales through multivariate forecasting.
The dummy data for this use case consists of multiple internal data sources, viz. warranty and financial management systems, joined together and hosted on a cloud data warehouse (AWS Redshift) as virtualized views or reflections.
The data’s schema consists of daily warranty claims data across 51 different attributes. The key attributes include:
- Key dimensions: Region, country, service center, product, model, warranty part family, causal part code, policy type, claim type, etc.
- Key measures: Warranty spending, part cost, labor cost, number of claims, etc.
- Date dimension: Credit issued date.
Descriptive analytics to help you explore the warranty spending data
Let us start analyzing warranty spending data for 2022. We will start with a simple descriptive analysis by asking the following question.
What was the warranty spending in 2022?
Image 1: Warranty spending in 2022
Lumin understood our question in natural language and converted it into an analytical query that included the time filter. As seen in Image 1, the warranty spending in 2022 is $ 30.77M. Now that we know the amount spent on servicing warranty claims in 2022, it would be interesting to understand its spread across the different operating regions. As a business, looking out for regions with significant warranty outlay is important.
What was the warranty spending across regions in 2022?
Image 2: Warranty spending in 2022 across regions. This Image also highlights a few features of Lumin viz., data export, chart switcher and connected insights.
Lumin allows us to:
- Export the underlying data
- Flexibility to switch the chart visualization
- Better navigate our insights exploration through the connected insights
As you can see in Image 2, Lumin dynamically selected the best-fit visualization to present the insight while generating a contextualized narrative (in English) to explain the same. It is evident that APAC has contributed to nearly half of the global warranty spending, which is interesting.
On clicking the connected insights button in Image 3, Lumin provides a set of simple and advanced analytical questions closely related to the metric or KPI we are exploring. As you can see in Image 3 below, Lumin has proactively nudged us on the increase in warranty spending for APAC in 2022 versus the year ago, which makes the case for APAC more intriguing. Similarly, we can explore other insights (and navigate to a detailed visualization and narrative) that Lumin has suggested.
Image 3: Connected insights
As the next logical step, it would be prudent to identify the countries within APAC that have had the highest share in warranty spending. We can ask Lumin the following question.
What was the share of warranty spending across Countries in APAC in 2022?
I would expect Lumin to show me a Pareto chart. It does exactly that. Lumin’s semantic model understands common business terminologies like ratio, share, contribution, top or bottom N, etc. It does not need to be trained separately on these. As is evident from Image 4, China leads the pack of countries within APAC in warranty spending. It has an almost 40% share.
Image 4: Pareto chart to depict the share of warranty spending across countries in APAC in 2022
Lumin provides us with some powerful collaboration features that allow us to:
- Share the insights with our colleagues within the organization .
- Add comments (our understanding, observations, POV, etc.) on the insights while we collaborate with a larger team.
- As seen in Image 4, one can create personalized alerts and signals to better track the metrics or KPIs, and get notified when they breach a threshold that we can define.
- Capture the insights in an insights storyboard called a workspace as we go about our exploratory journey. The workspace can further be shared with our colleagues within the organization.
Since we know China had the highest share, the next obvious question would be: Has warranty spending grown M-O-M in 2022 or were there certain months where we have seen an unexpectedly high spending? To answer this, we can ask Lumin.
What was the monthly growth of warranty spending in China in the last 12 months?
Image 5: M-O-M growth in warranty spending in China in 2022
The insight in Image 5 suggests that the warranty spending has oscillated in the $300K to $ 700K range. However, a few months, like June or October, witnessed a sudden increase in warranty spending compared to the previous month. As per Lumin’s Natural Language Generation (NLG) narrative, the highest M-O-M rise was witnessed in October 2022.
With this information, wouldn’t it be interesting to know which cities within China contributed the most to that increase in warranty spending in October 2022? Let us ask Lumin.
What contributed to the growth of warranty spending in China across cities in Oct 2022 vs. Sep 2022?
Image 6: Cities contributing to the growth of warranty spending in China in October 2022
As evident from the waterfall chart in Image 6, Shanghai, Shenzhen, and Tianjin have driven the increase in warranty spending in China in October 2022. Shanghai leads the pack with an almost 2/3rd increase in October vs. the previous month.
Never miss a critical change in your warranty spending with autonomous nudges
We can continue even further and ask even more questions. We can probe the data for more deep-dive analyses across various dimensions. We began our analysis by looking at APAC’s geographical cut. Beyond this, we can look at products and models, policy types, claim types, etc., and arrive at granular insights enabling increased confidence in enterprise decision-making.
Still, what if Lumin could have autonomously detected and nudged us on anomalistic trends? Could this have been possible? Yes, Lumin can spot deviations in our data as they happen with fully automated ‘nudges.’ Lumin’s anomaly engine synthesizes millions of data points in seconds to inform us of important changes as they happen. It detects what had shifted, when, why this occurred, and by how much.
In this sample use case, we set up autonomous nudges on warranty spending. We configured it to look for anomalistic behavior in warranty spending across the geographical hierarchy comprising regions, cities, and service centers. Lumin proactively detected the sudden increase in warranty spending in APAC-China-Shanghai, as seen in Image 7. It also pointed to the fact that an increase led to the growth in labor. The growth was witnessed particularly at account 10 (AN10) in Shanghai.
Image 7: Autonomous nudge detected the unexpected increase in warrant spending in Shanghai, China in October 2022
As you can see in Image 7, autonomous nudges, leveraging machine learning, proactively surfaced the anomaly for which we had to otherwise ask multiple questions on Lumin.
Go beyond insights to reveal the “why” and “what if”
We discussed earlier that one of the ways to analyze the increase in warranty spending in China is to cut the metric across various dimensions and hierarchies. This will require us to ask multiple questions and take a lot of time. This problem makes the process inefficient. However, we can diagnose the reason for the increase by asking a single “Why” question to Lumin.
Why did the warranty spending for China increase in October 2022 vs. PP?
Image 8: Reasons for the increase in warranty spending in China in October 2022
As seen in Image 8, Lumin performs a cross-dimensional analysis of warranty spending. This analysis included the:
- Product hierarchy (product, model)
- Policy hierarchy (policy type, claim type)
- Warranty part hierarchy (part family, part code)
- The geographical hierarchy that we have already analyzed
In a matter of a few seconds, Lumin presents a detailed narrative and an interactive bubble chart that illustrates the deep-dive analysis across multiple levels like geography, product, policy, and warranty.
For example, if we analyze from a product standpoint, we understand that the Iceulite model contributed the most to the increase in warranty spending. The warranty claims for compressors with the part number 02-87101-81 drove it. Also, for Iceulite, the warranty spending was the highest in Shanghai, led by account 10 (AN 10).
Now, as users, we can very well question these findings. Enter, Lumin’s important explainability layer. It explains, as seen in Image 9:
- How it arrived at these insights.
- What was the input data.
- The sanity of the applied model, its reliability, accuracy, etc.
Image 9: Insights explainability
Apart from answering the “Why” question, Lumin provides us with a list of drivers (and their performance) that impact warranty spending based on the correlation matrix that Lumin autonomously creates under its hood.
As showcased in Image 10, Lumin has identified Labor costs as a significant (and sole) driver impacting warranty spending for Iceulite. You can explore the drivers for some other significant dimensions like compressors, Shanghai, concession policy, and extended coverage policy. These contributed to the increase in warranty spending for China in October 2022.
Image 10: Key driver analysis
Now that we know the drivers for warranty spending, we can simulate the same for China by changing the drivers, i.e., reducing the Labor Cost by 40%, as illustrated in Image 11.
Image 11: Simulation – Changing the drivers for simulation
Image 12: Simulation
As shown in Image 12, a 40% reduction in labor costs further reduces warranty spending by 13%. We can click on the “How did I arrive at the Insight” link to understand the computation Lumin performed to simulate the results.
Get a sneak peek into the future warranty spending using predictive analytics
Lastly, given the historical trend, it will be crucial to understand how warranty spending will trend in China over the next few months. This will help the business to be better prepared (in terms of reserves) to manage a possible increase in spending. Let us ask Lumin to forecast the warranty spending for China.
What will be the warranty spending for China in the next three months?
Image 13: Forecast of warranty spending in China
Image 14: Explainability of forecast
Lumin understands that we are asking it to predict the warranty spending for China. In turn, it runs multiple multivariate forecasting models under the hood and presents the most accurate model as an output. This is demonstrated in Image 13. For our question, Lumin selected the multivariate time series ARIMAX model for predicting warranty spending, and it also identified the part cost and labor cost as the key drivers impacting the forecast.
As before, to usher in trust and transparency, Lumin provides a detailed explanation of the input data, its sanity, the prediction models it applied (including accuracy), how we interpret them, and the reliability of those models – as shown in Image 14.
However, as a business, if we are aware that the part cost and labor costs are expected to increase in the next three months, it would be vital for us to factor that increase into our forecast to make realistic plans. Since we know that part and labor costs impact warranty spending in China, let us increase them to $150K and $25K in the next three months and simulate the output. This strategy is highlighted in Image 15.
Image 15: Forecast simulation – updating the drivers for warranty spending for the simulation
Image 16: Forecast simulation
As you can see, Lumin factored in the increase in the drivers and reworked the forecast for us, as seen in Image 16. The forecasted values have increased compared to the earlier forecast. This is significantly more realistic and closer to what the business would expect.
Create, curate, and collaborate on your insight story
The purpose of the entire analysis we performed as part of this blog post is not to ask questions but to tell an insightful data story that can be shared with the relevant stakeholders across the organization. Lumin, through the workspace storyboards, enables us to seamlessly create, curate and collaborate with our colleagues in the organization.
Image 17: Workspace storyboard
As we traverse through our insights exploration journey, we can create a personalized workspace, as showcased in Image 17, and add our insights to that workspace. Once the analysis is complete, we can visit our workspace to refine or curate our story, add comments, etc., before publishing it to the stakeholders. Once published, the workspace is refreshed every time the underlying data is refreshed. Isn’t that amazing?
Lumin: The warranty analytics solution
As we showcased above, Lumin delivers value across the manufacturing industry’s after-market sales and service value chain by providing finance, quality, and service engineering teams with a unified analytics experience across data sources. It can curate faster answers to what happened with a Google-like conversational interface. It explains business metric changes and how to improve business outcomes by understanding the drivers of those behaviors.
Learn more with us at Lumin by Fosfor.