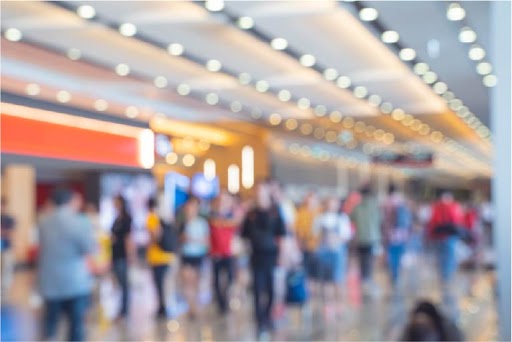
Introduction
In today’s fast-paced digital landscape, the retail sector is undergoing a profound transformation. With the rise of e-commerce and omnichannel retailing, the need for robust security measures has never been more urgent. Retailers now face a wide array of threats, ranging from cyber-attacks and data breaches to sophisticated fraud schemes. To address these challenges, Generative AI (GenAI) is emerging as a game-changer, offering innovative solutions that help build a secure retail ecosystem. This article delves into how GenAI can revolutionize fraud detection and enhance security within the retail industry.
Understanding GenAI and its potential
Generative AI, or GenAI, refers to AI systems that can generate new data based on existing information, making it a powerful tool in detecting anomalies, predicting fraud, and enhancing security. By leveraging advanced machine learning algorithms, GenAI can analyze vast datasets in real-time, identify patterns, and respond proactively to potential threats. Retailers adopting this technology are poised to deliver a more secure, trustworthy, and efficient shopping experience for both themselves and their customers.
The growing threat landscape in retail
The retail industry is a prime target for cybercriminals due to the vast amounts of sensitive customer data it handles, including payment information, personal details, and purchasing habits. Common threats include:
- Payment fraud: Unauthorized transactions and the use of stolen credit card information.
- Account takeover: Cybercriminals gaining access to customer accounts to make unauthorized purchases.
- Data breaches: Unauthorized access to sensitive customer and company data.
- Return fraud: Manipulating the return process to exploit the retailer.
How GenAI enhances fraud detection
Generative AI in retail revolutionizes fraud detection by enabling real-time data analysis and identifying subtle patterns indicative of fraudulent activity. Below are several powerful ways GenAI enhances fraud detection:
1. Cutting-edge anomaly detection
Example: A retail company sees a sudden spike in high-value transactions from a specific customer account. Traditional systems might miss this, but GenAI can learn from historical data to spot unusual patterns.
How it works:
- Data analysis: Continuously evaluates transaction data to understand typical customer behavior.
- Pattern recognition: Identifies anomalies that deviate from established patterns, such as numerous transactions in a short period.
- Alert generation: Generates alerts for further scrutiny when an anomaly is detected.
- Outcome: Early fraud detection, allowing the retailer to swiftly prevent financial loss.
2. In-depth behavioral analytics
Example: An online retailer notices multiple login attempts to a customer’s account from different locations within a short time. Traditional systems might not flag this if each attempt uses the correct credentials.
How it works:
- User profiling: Creates detailed profiles of customer behavior, including typical login times and locations.
- Deviation detection: Spots deviations, such as logins from unfamiliar locations or devices.
- Real-time alerts: Generates real-time alerts for suspicious activity, prompting additional verification.
- Outcome: Reduced risk of account takeover fraud, bolstering customer trust and security.
3. Synthetic data generation for enhanced training
Example: A small e-commerce startup needs to train its fraud detection model but lacks sufficient historical fraud data.
How it works:
- Data creation: Generates synthetic data mimicking real-world fraud scenarios.
- Model training: Uses synthetic data to train the fraud detection model, ensuring it can recognize a wide range of fraud patterns.
- Testing and validation: Tests and validates the model with synthetic data to ensure accuracy and robustness.
- Outcome: Improved fraud detection capabilities without compromising sensitive customer information.
4. Credit card fraud mitigation
Example: A major bank integrates GenAI into its fraud detection system to monitor credit card transactions.
How it works:
- Transaction monitoring: Monitors transactions in real-time, assessing factors such as amount and customer location.
- Risk scoring: Assigns a risk score to each transaction.
- Automated response: Flags or declines high-risk transactions.
- Outcome: Decreased instances of credit card fraud, protecting both the bank and its customers.
5. E-commerce platform security
Example: An e-commerce platform uses GenAI to detect fraudulent activities like fake accounts and fraudulent listings.
How it works:
- User activity analysis: Analyzes user activities, including account creation patterns and transaction histories.
- Anomaly detection: Identifies unusual activities, such as multiple accounts from a single IP address.
- Proactive measures: Flags or removes suspicious accounts and listings.
- Outcome: Enhanced platform security, reducing the risk of fraud.
6. Point-of-sale (POS) fraud detection
Example: A retail chain uses GenAI in its POS systems to detect fraud at checkout.
How it works:
- Transaction analysis: Analyzes each transaction for patterns indicative of fraud, such as high discounts or frequent returns.
- Employee monitoring: Monitors employee behavior to identify potential internal fraud.
- Immediate alerts: Triggers alerts for suspicious transactions.
- Outcome: Improved detection of POS fraud, protecting revenue.
Challenges and considerations in implementing GenAI for fraud detection
While GenAI presents a promising solution for enhancing security and fraud detection in retail, its implementation brings several challenges:
1. Data quality and quantity
Challenge: GenAI requires vast amounts of high-quality data to function effectively. Inaccurate or insufficient data can lead to poor model performance.
Consideration: Ensure data is clean, comprehensive, and representative of various fraud scenarios. Smaller retailers might need to use synthetic data to augment their datasets.
2. Privacy concerns
Challenge: Collecting and analyzing customer data can raise privacy issues and regulatory compliance challenges.
Consideration: Implement strict data governance policies and ensure compliance with relevant data privacy regulations to protect customer information and maintain trust.
3. Integration with existing systems
Challenge: Integrating GenAI with existing IT infrastructure and legacy systems can be complex and costly.
Consideration: Conduct a thorough assessment of current systems and plan for gradual integration, ensuring compatibility and minimizing disruption to operations.
4. False positives and negatives
Challenge: GenAI models can produce false positives (legitimate transactions flagged as fraud) and false negatives (fraudulent transactions not detected).
Consideration: Continuously monitor and refine the GenAI model to balance sensitivity and specificity, reducing the occurrence of false positives and negatives.
5. Cost and resource investment
Challenge: Developing and maintaining GenAI systems requires substantial financial and technical resources.
Consideration: Evaluate the cost-benefit ratio and consider phased implementation or leveraging third-party GenAI solutions to manage costs effectively.
6. Ethical considerations
Challenge: Ensuring that AI systems operate ethically and do not inadvertently reinforce biases.
Consideration: Regularly audit AI systems for bias, ensure diverse training data, and establish ethical guidelines for AI usage.
Conclusion
The integration of GenAI in the retail ecosystem represents a significant leap forward in the fight against fraud and security breaches. GenAI’s capabilities in real-time data analysis, adaptive learning, and predictive analytics empower retailers to detect and mitigate threats with unprecedented accuracy and efficiency. By enhancing fraud detection measures, securing sensitive data, and maintaining customer trust, GenAI transforms the retail landscape into a more secure and resilient domain.
As retailers continue to navigate the complexities of digital transformation, adopting GenAI is not merely an option but a necessity. The stakes are high, with both financial and reputational implications hinging on the effectiveness of fraud detection and security strategies. By investing in GenAI, retailers position themselves to not only protect their assets but also to foster customer loyalty and drive business growth.
Looking ahead, the potential of GenAI in retail security is boundless. As the technology continues to evolve, it will unlock new avenues for innovation, setting new standards for what a secure and seamless retail experience should be. Retailers who embrace this technological advancement will be well-equipped to face the challenges of tomorrow, ensuring a safe and trustworthy shopping environment for their customers. The path forward is clear—embrace GenAI and build a secure future in retail.