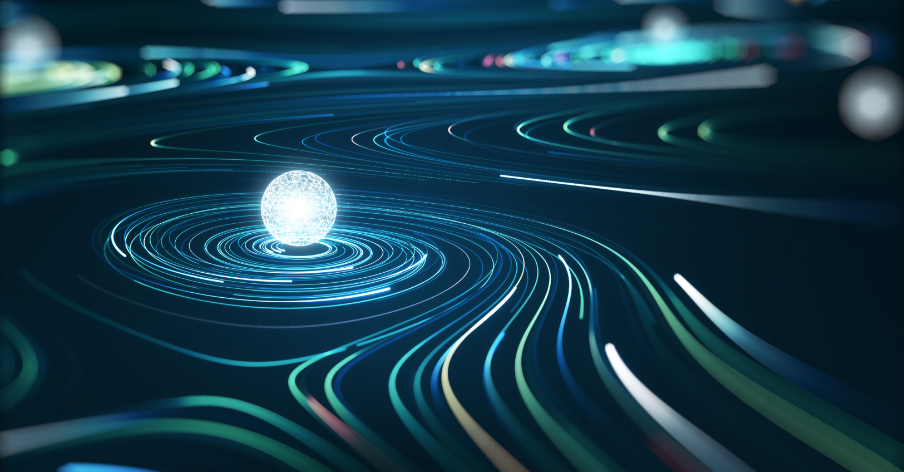
Introduction
With the ever-increasing volume and complexity of data, extracting value from it has become more challenging. Organizations are facing challenges in providing the right set of data to the right team while maintaining data security at the same time. This presents an opportunity for organizations to become agile with their data management solution, provide the right data set to the correct domain team, and ensure collaboration among teams to get the maximum out of their data.
Forrester defines Data Operations (DataOps) as “the ability to enable data management solutions, develop data products, and activate data for business value across all technology tiers from infrastructure to experience.”
DataOps provides the perfect opportunity to create data management solutions to improve communication, integration, and automation of data flow between managers and consumers of data within an organization. We will try to understand how we look at modern DataOps in the Enterprise Data Analytics ecosystem through this blog.
What is DataOps
Gartner defines DataOps as “a collaborative data management practice focused on improving the communication, integration and automation of data flows between data managers (Data Engineers, Data Architects, Data Stewards) and data consumers (Data Scientists, Business Analysts, Business teams) across an organization.”
Effectively, DataOps seeks to provide the tools, processes, and organizational structures to effectively fulfill this significant expectation from modern data enterprises.
What’s vital for us to understand here is the core data management solution for DataOps — Agility. Agility refers to dynamism primarily for data consumers in a conventional data enterprise who need an enterprise DataOps platform to be quick to react in a data-driven way to their business needs.
Modern-day DataOps prescribes the use of technology to bring in higher levels of data automation across the lifecycle of a data project or data product — from concept, development, and deployment to ongoing operational monitoring. It even caters to the last-mile data delivery to consumers. By optimizing an enterprise’s data and analytics value chain, distributed domain-centric data teams can build their own data products and achieve higher efficiency and unparalleled agility in conducting their day-to-day data management.
The evolution
‘What is your strategy for the enterprise data analytics process?‘
This is a question that any C-suite executive is comfortable answering today. In fact, the existence of many data-driven organizations depends on effectively answering this question. But this wasn’t always the case. From being an afterthought to being the necessary hygiene to being the key driver for business success, we have witnessed an impressive transition for data organizations. Organizations implementing the enterprise DataOps platform have seen a sea of change in how they are positioned in context to the business in the last couple of decades. Figure 1 outlines this information as well.
The early 2000s: The key driver for data organizations in the early 2000s was record keeping. For continuity and compliance, distributed business applications were needed to centralize data in a warehouse. Consumption of such centrally stored data wasn’t much of a concern for the enterprises. Data orchestration was the flavor of the season for any data tools and platform during this era.
The 2010s: The last decade has seen enterprises realize the potential in their enterprise data assets. To that end, they scrambled towards doing even more centralized storage, adding new data varieties like unstructured or semistructured content at peculiar velocities and unprecedented volumes. With all such data in one place, the next important step was to enforce more elaborate access controls and governance. At this point, we saw a sharp focus on tooling for setting up data lakes, curating purpose-built data marts, enforcing granular data governance and security, lineages etc.
While these still are fundamental components of an enterprise data ecosystem, the whole approach of centrally owned and managed data estate was in for a total shake-down soon.
The 2020s: Today’s businesses hold data-driven decision-making at the core of their business model. For the first time, this has translated into increased demand for very high visibility and access to enterprise data assets across an organization.
The resounding focus now rests on agility and independence. Enterprises want to serve the data to consumers so that distributed departmental teams can use it in their business decisions workflows. To this end, modern data management platforms demand:
- More democratized access to data
- Easy to use and low code design interfaces
- Higher domain contextualization for distributed departmental teams.
These three components need to work well together while adhering to set security and governance practices.
The paradigm shift is from a centrally owned and managed data estate to a decentralized and federated data ecosystem. This, of course, will call for higher collaboration, contextualization and coverage for today’s data organizations.