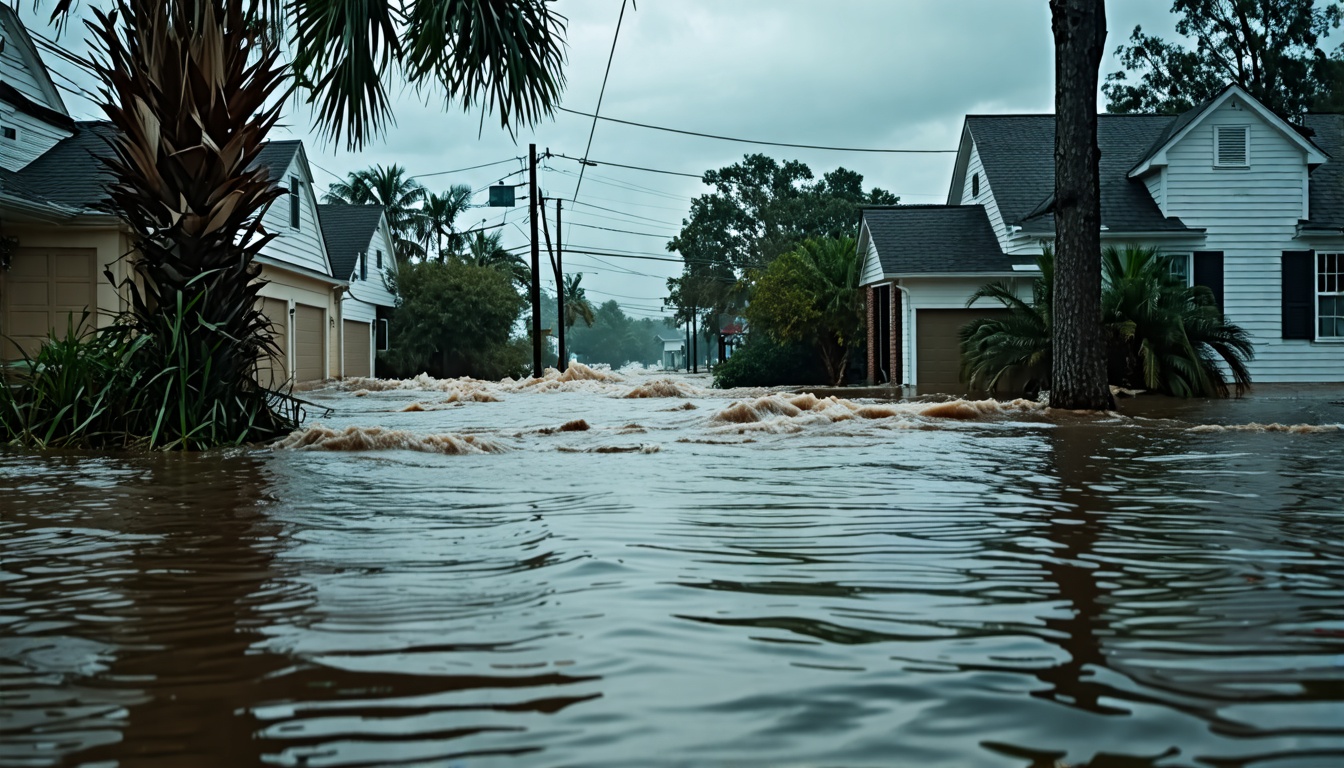
The property and casualty insurance industry faces constant challenges, especially with the increasing frequency and severity of natural disasters such as hurricanes. Flood risks caused by hurricanes present a unique challenge for underwriters assessing and managing risk effectively. Traditionally, underwriting flood risks have involved labor-intensive processes, subjective decision-making, and lengthy evaluation periods. However, with the integration of AI and decision intelligence, insurers can transform these processes, enhancing efficiency and accuracy. This blog explores how AI and decision intelligence improve operational efficiency in underwriting by automating risk assessments, processing data intelligently, and providing targeted risk mitigation strategies for flood-prone properties.
Automated risk assessment and segmentation
One of AI’s primary benefits in underwriting is the ability to automate risk assessment and segmentation. AI models analyze structured data, such as historical claims data, property characteristics, and environmental factors, to generate accurate property risk scores. For instance, properties in hurricane-prone areas can be automatically scored based on their distance from the coast, elevation, and construction materials, allowing insurers to categorize properties into different risk tiers.
Example
Consider historical claims data that includes the frequency and severity of flood damage in various locations. AI models can analyze this data to determine which properties are at higher risk of flooding due to hurricanes. Properties in high-risk areas are flagged for further review, while those with lower-risk scores can be automatically processed with minimal human intervention.
Operational efficiency created
- Faster segmentation: Automated property categorization speeds up the underwriting process, enabling insurers to quickly identify high-risk properties.
- Reduced manual reviews: By allowing AI to handle low-risk cases, underwriters can focus their attention on complex, high-risk cases, significantly reducing manual review time.
- Consistent risk scoring: AI ensures uniformity in risk evaluations, reducing variability in decisions caused by human subjectivity.
Intelligent document processing
Underwriting often involves reviewing many documents, such as inspection reports, policy applications, and flood zone maps. Traditionally, these documents require manual data entry and verification, which is time-consuming and prone to errors. AI-powered Optical Character Recognition (OCR) and Natural Language Processing (NLP) technologies can automatically extract and validate data, streamlining document processing.
Example
When underwriters receive inspection reports detailing flood defenses like levees or flood doors, AI models can extract relevant data points and cross-reference them with updated flood zone maps. This process ensures that underwriting decisions are based on the latest information, enhancing accuracy.
Operational efficiency created
- Reduction in data entry errors: Automated data extraction ensures that information is processed accurately, minimizing the risk of human error.
- Accelerated decision-making: AI reduces document processing time from days to minutes, allowing underwriters to make quicker, data-driven decisions.
- Improved data consistency: AI validation against external sources ensures that all data used in underwriting is accurate and up to date.
Predictive analytics for loss prevention
Predictive analytics is a powerful tool in the underwriting process, especially when anticipating flood risks. By analyzing structured data such as historical hurricane paths, rainfall data, and past flood claims, AI models can forecast the likelihood and potential severity of future flood events. This proactive approach allows insurers to adjust premiums, modify coverage terms, or recommend loss prevention measures before a loss occurs.
Example
AI models can predict the probability of a hurricane impacting a specific area based on historical weather patterns and climate data. If the model forecasts an increased likelihood of severe flooding, underwriters can adjust the terms of coverage for properties in the affected areas, such as requiring additional flood defenses or increasing deductibles.
Operational efficiency created
- Proactive adjustments: Underwriters can make data-driven adjustments to policies before a disaster occurs, reducing the frequency and severity of claims.
- Enhanced predictive accuracy: AI-driven models provide accurate forecasts, enabling insurers to better price risks and mitigate potential losses.
- Fraud reduction: Predictive models can also identify anomalies and patterns indicative of fraudulent claims, reducing the overall cost of claims.
Personalized underwriting strategies
AI enables the creation of personalized underwriting strategies by leveraging structured data from customer profiles, property characteristics, and past claims. This allows insurers to tailor policies to individual risk profiles, enhancing customer satisfaction and retention. AI models can provide targeted recommendations for policy adjustments based on specific property details, such as previous flood claims or installed mitigation measures.
Example
If a customer has installed flood barriers or made other improvements to reduce their property’s flood risk, AI can adjust the underwriting strategy to offer more favorable terms. This not only incentivizes customers to take proactive measures but also helps insurers manage their overall risk exposure.
Operational efficiency created
- Tailored coverage: Personalized policies reflect each property’s unique risk profile, improving coverage and risk alignment.
- Improved risk management: AI-driven recommendations encourage customers to adopt flood prevention measures, reducing the likelihood of future claims.
- Increased customer retention: Offering personalized and responsive underwriting strategies builds stronger customer relationships.
Workflow automation and decision support
Workflow automation using AI and robotic process automation (RPA) significantly reduces the administrative burden on underwriters. By automating repetitive tasks, such as data entry, policy renewals, and compliance checks, AI enables underwriters to focus on high-value decision-making. AI-powered decision trees guide underwriters through complex decisions, ensuring that all underwriting guidelines are consistently applied.
Example
RPA can automate integrating quote data, inspection outcomes, and flood zone classifications into underwriting systems, updating policies in real-time as new information becomes available. This ensures that underwriters always work with the most current data.
Operational efficiency created
- Streamlined processes: Automation of routine tasks reduces the time spent on administrative duties, freeing underwriters to concentrate on more complex cases.
- Consistency in decision-making: AI ensures that underwriting guidelines are applied uniformly, reducing inconsistencies and manual errors.
- Faster customer response times: Automated workflows mean quicker responses to customer inquiries and faster policy issuance.
Real-time data integration and analysis
AI’s ability to integrate and analyze real-time data is critical in managing hurricane flood risks. Real-time weather data, flood alerts, and updated floodplain maps provide underwriters with the most current risk information, enabling them to make timely adjustments to coverage and pricing.
Example
During hurricane season, AI systems continuously monitor weather data and flood alerts, automatically updating risk assessments for properties in the projected path of a storm. This allows underwriters to take immediate action, such as adjusting coverage limits or recommending additional safety measures.
Operational efficiency created
- Rapid response to changing conditions: Real-time data integration enables immediate adjustments to underwriting decisions based on the latest information.
- Reduced decision latency: Access to current data accelerates the decision-making process, ensuring that underwriting remains relevant and timely.
- Improved risk visibility: Continuous data updates provide a clearer view of evolving risks, supporting more accurate underwriting.
Continuous learning and model updates
AI models are only as good as the data they are trained on, so continuous learning and regular updates are essential. AI models can refine their predictions and stay aligned with current trends by integrating feedback loops from claims data, updated hurricane forecasts, and market conditions.
Example
AI models are retrained with new data from recent hurricane seasons, incorporating changes in weather patterns, building practices, and property maintenance trends. This ensures flood risk assessments remain accurate and reflect the latest environmental factors.
Operational efficiency created
- Adaptive risk models: Continuous updates ensure that models evolve with new data, maintaining up-to-date risk assessments.
- Reduced manual model management: Automated retraining of models eliminates the need for manual updates, saving time and ensuring relevancy.
- Enhanced predictive power: Regularly updated models provide more reliable forecasts, supporting better underwriting decisions.
Enhanced customer communication and support
AI-driven chatbots and decision support systems enhance customer communication by providing instant responses to inquiries about flood preparedness and insurance needs. These systems analyze structured data from customer interactions, past claims, and policy adjustments to offer tailored advice and proactive communication.
Example
AI chatbots can handle customer queries regarding flood risk, coverage options, and steps to mitigate potential damage. The chatbot can also proactively reach out to customers in high-risk areas ahead of hurricane season, recommending adjustments to coverage or highlighting necessary flood defenses.
Operational efficiency created
- Faster customer support: Automated responses reduce the need for underwriter involvement in routine communications, freeing resources for more complex issues.
- Proactive engagement: AI-driven communication helps keep customers informed and prepared, enhancing their overall experience.
- Increased conversion rates: Timely, data-driven communication improves the likelihood of policy renewals and upsells.
Explainable AI for regulatory compliance
Compliance is a critical component of underwriting, especially when managing flood risks. Explainable AI provides transparent reports detailing how decisions are made, including data inputs, model logic, and decision paths. This ensures that underwriting decisions are not only accurate but also fully compliant with regulatory requirements.
Example
Structured decision logs capture the steps taken during underwriting, including the rationale behind risk assessments and coverage adjustments. This data is readily available for audits, simplifying compliance checks and providing a clear trail of accountability.
Operational efficiency created
- Simplified compliance audits: Detailed logs reduce the time required for audits, as decision-making processes are clearly documented.
- Reduced compliance risk: Transparent decision-making minimizes the risk of regulatory penalties, ensuring that all underwriting actions are fully compliant.
- Increased trust in AI systems: Explainable AI builds confidence among regulators, underwriters, and customers.
Risk mitigation and risk improvement interventions for high-risk and frequent loss properties
AI enables insurers to identify high-risk and frequently affected properties and recommend targeted risk mitigation and improvement interventions. This proactive approach helps insurers not only manage current risks but also prevent future losses by guiding policyholders toward actionable improvements.
Example
AI models analyze claims data, property inspection reports, and environmental patterns to identify properties frequently affected by floods. For these high-risk properties, the system generates specific mitigation recommendations, such as installing flood barriers, improving drainage systems, elevating utilities, or using water-resistant building materials. Insurers can provide policyholders with suggested actions, often incentivized by premium reductions upon completion.
Operational efficiency created
- Proactive risk management: AI-driven insights allow insurers to intervene before losses occur, encouraging policyholders to adopt preventative measures that reduce the likelihood of future claims.
- Reduction in loss frequency and severity: Targeted mitigation efforts directly reduce the number and impact of claims, improving the insurer’s loss ratio and overall portfolio health.
- Enhanced customer support and trust: By providing data-backed recommendations and engaging policyholders in risk improvement, insurers position themselves as proactive partners rather than just service providers. This can lead to higher customer satisfaction and loyalty.
- Optimized underwriting decisions: As properties undergo recommended improvements, underwriters can adjust policy terms and premiums to reflect the reduced risk, allowing for more competitive pricing and better alignment between coverage and risk.
Conclusion
Integrating AI and decision intelligence into the underwriting function of property and casualty insurance offers transformative benefits, especially when managing complex risks like those posed by hurricanes and floods. From automated risk assessment and predictive analytics to real-time data integration and targeted risk mitigation strategies, AI enables insurers to streamline processes, enhance accuracy, and proactively manage risk.
These efficiencies not only reduce operational costs and improve underwriting speed but also position insurers to offer more tailored, responsive, and competitive products in an increasingly dynamic market. By embracing these advanced technologies, insurers can move beyond traditional methods and achieve a higher level of precision, agility, and customer-centricity in their underwriting processes.
As climate change continues to increase the frequency and severity of natural disasters, the need for innovative solutions in underwriting becomes even more critical. AI and decision intelligence provide insurers with the tools needed to not only adapt to these challenges but to thrive in the face of them, ensuring better protection for policyholders and more sustainable business outcomes for insurers.
How the Fosfor Decision Cloud can help
The Fosfor Decision Cloud offers a robust platform that integrates AI, machine learning, and decision intelligence to help insurers realize these operational benefits. By leveraging the Fosfor Decision Cloud, insurers can harness real-time data, predictive analytics, and automated decision-making to enhance underwriting precision, streamline workflows, and drive proactive risk management. Its capabilities in data integration, model management, and explainable AI ensure that underwriting decisions are not only faster but also compliant and transparent. With the Fosfor Decision Cloud, insurers can transform their underwriting operations, achieving greater efficiency, reducing costs, and providing superior value to their customers in an ever-evolving risk landscape.