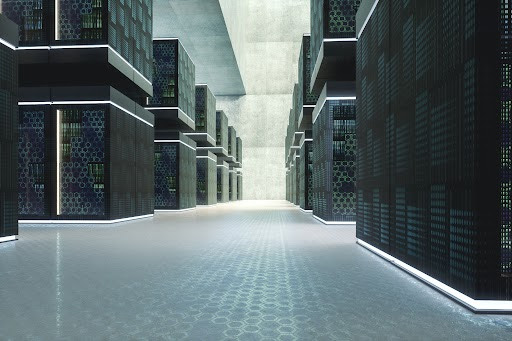
Introduction
As the digital landscape continues to expand and evolve, the challenges within the modern data stack have become increasingly complex. The need to process, store, and analyze vast amounts of data has given rise to new trends and technologies that are shaping the way organizations manage their data. In this blog, we will explore the top 5 trends in relation to the modern data stack that are redefining the way data is collected, stored, and utilized.
These trends have emerged as a response to the growing demands for faster, more efficient, and more cost-effective data management solutions, and they are reshaping the way organizations approach data analytics and decision-making.
1. Cloud-native data warehousing/Data lakes take center stage
The shift towards cloud-native data warehousing solutions, such as Snowflake and Databricks, is driven by the scalability, flexibility, and cost-effectiveness offered by cloud platforms. Organizations are leveraging cloud-native data warehouses to store and analyze massive volumes of data, enabling real-time analytics and decision-making. This trend is reshaping traditional data warehousing approaches and empowering organizations to rethink their approach to the modern data stack.
The fast-growing adoption of the Snowflake Native App Framework (which is now generally available on AWS and Azure, private preview on GCP), tells us that native app building for enterprises is here to stay.
As organizations continue to embrace cloud-native data warehousing solutions, it is becoming increasingly important to ensure the security and privacy of sensitive data. This has led to a growing focus on implementing robust data governance and compliance measures within cloud data warehouses, further enhancing the value and trustworthiness of these platforms – the modern data stack is at the heart of this change.
2. DataOps and MLOps converge to enhance operational efficiency
The convergence of DataOps and MLOps practices is transforming the way organizations manage and deploy data and machine learning models. This trend emphasizes the integration of data engineering, data governance, and machine learning lifecycle management, enabling seamless collaboration and automation across the data and AI pipeline, symbolized by the modern data stack.
This convergence also highlights the growing importance of cross-functional teams and the need for a unified approach to data and machine learning operations. By breaking down silos and promoting collaboration, organizations can accelerate innovation and drive better business outcomes.
3. Data privacy and governance have become the norm
Amid increasing regulatory scrutiny and data privacy concerns, organizations prioritize data privacy and governance as a fundamental trend in setting up the modern data stack. Strong data governance means the organization has adequate safeguards to ensure high data observability, easy data discovery, and reliable data privacy and security. Technologies such as HashiCorp Vault and Amazon Macie are empowering organizations to secure sensitive data, enforce data access controls, and comply with privacy regulations such as GDPR and CCPA. This trend is essential for building trust and transparency in data management practices as enterprises set up their modern data stack.
As organizations continue to expand globally, they also face the challenge of navigating a complex web of international data privacy laws and regulations. This has led to an increased focus on developing cross-border data transfer mechanisms and ensuring compliance with region-specific privacy requirements, further highlighting the importance of data privacy and governance in the modern data stack.
4. Generative AI aligned with the modern data stack
The intersection of the modern data stack and Generative Artificial Intelligence presents a fascinating opportunity to revolutionize data-driven decision-making and innovation. Generative AI, fueled by advancements in deep learning and neural networks, is revolutionizing data generation, synthesis, and augmentation within the modern data stack. Gen AI algorithms can leverage the rich datasets stored in modern data warehouses to generate predictive models and insights. Gen AI algorithms powered by NLP techniques can analyze unstructured textual data stored in data lakes or document repositories.
By combining the scalability and flexibility of modern data infrastructure with the cognitive capabilities of Gen AI, organizations will look to create data stacks that can help them gain deeper insights, drive operational efficiencies, and create more personalized and impactful experiences for their customers.
5. Bundling of the modern data stack’s components for simplicity
As businesses continue to expand their data infrastructure with new tools and technologies to keep pace with evolving data requirements, they often encounter complexities in integration, maintenance, and optimization. The addition of each new component introduces potential points of failure, exacerbating the risk of system inefficiencies, data inconsistencies, and security vulnerabilities. Furthermore, demonstrating the return on investment (ROI) for the existing data stack presents a significant hurdle for enterprises.
Consolidation of the modern data stack is becoming increasingly relevant as businesses seek to streamline their data infrastructure, reduce complexity, and improve efficiency. As organizations continue to prioritize data-driven decision-making, the demand for a more efficient and integrated data stack will only continue to grow. This trend underscores the importance of leveraging advanced technologies and platforms to create a modern data stack that can unlock the full potential of data to drive business success. The Fosfor Decision Cloud exemplifies this trend by offering a unified solution for data integration, governance, and insights generation.
Future outlook
Looking ahead, the modern data stack is poised to undergo further transformation and innovation. The integration of AI and machine learning into every aspect of data management, the proliferation of edge computing for real-time data processing, and the continued evolution of data governance and ethics are anticipated to be key drivers of change. As organizations embrace a data-centric culture, the modern data stack will continue to evolve to meet the demands of an increasingly complex and dynamic data landscape.